How to Start Modeling: Essential Tips to Succeed in 2025
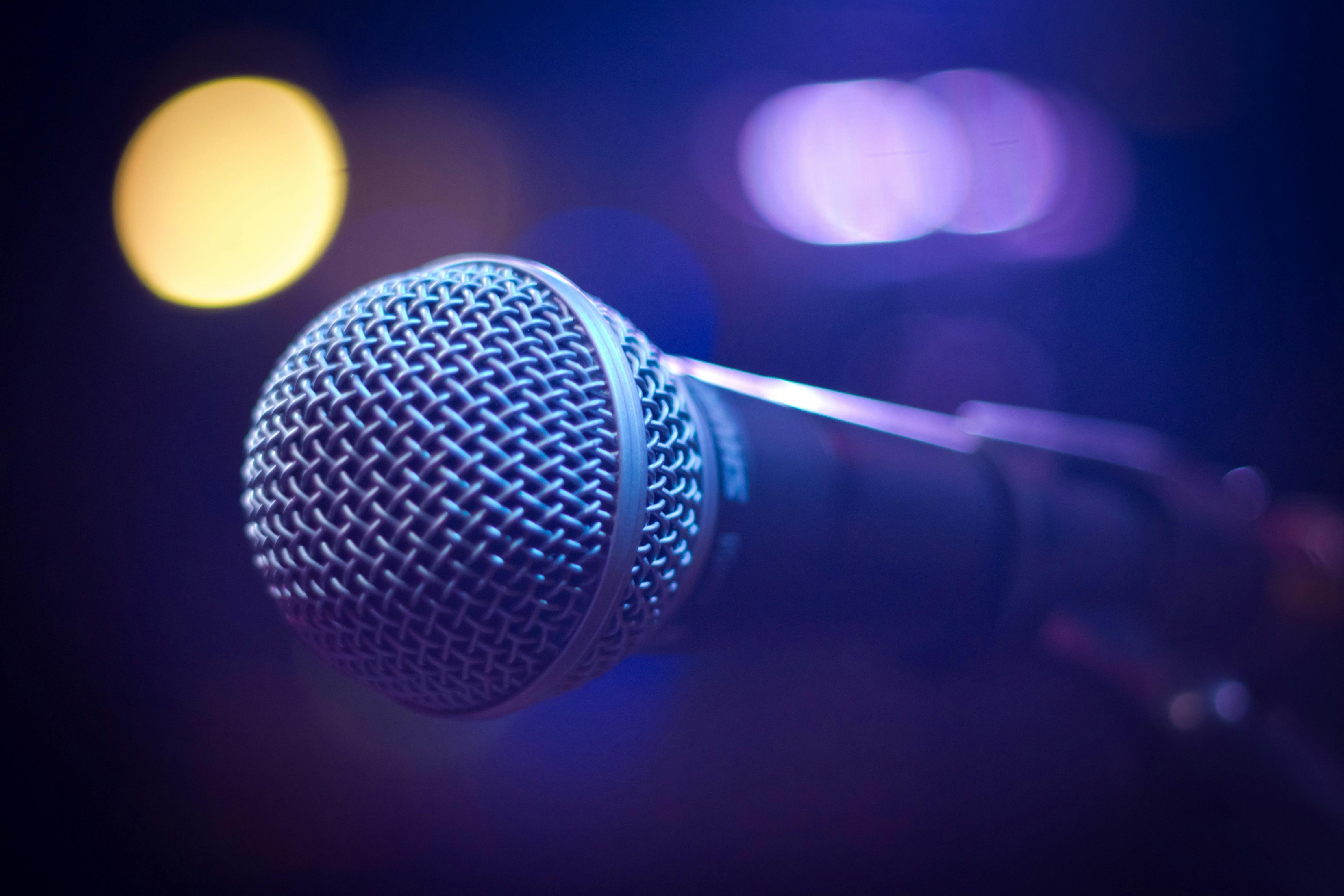
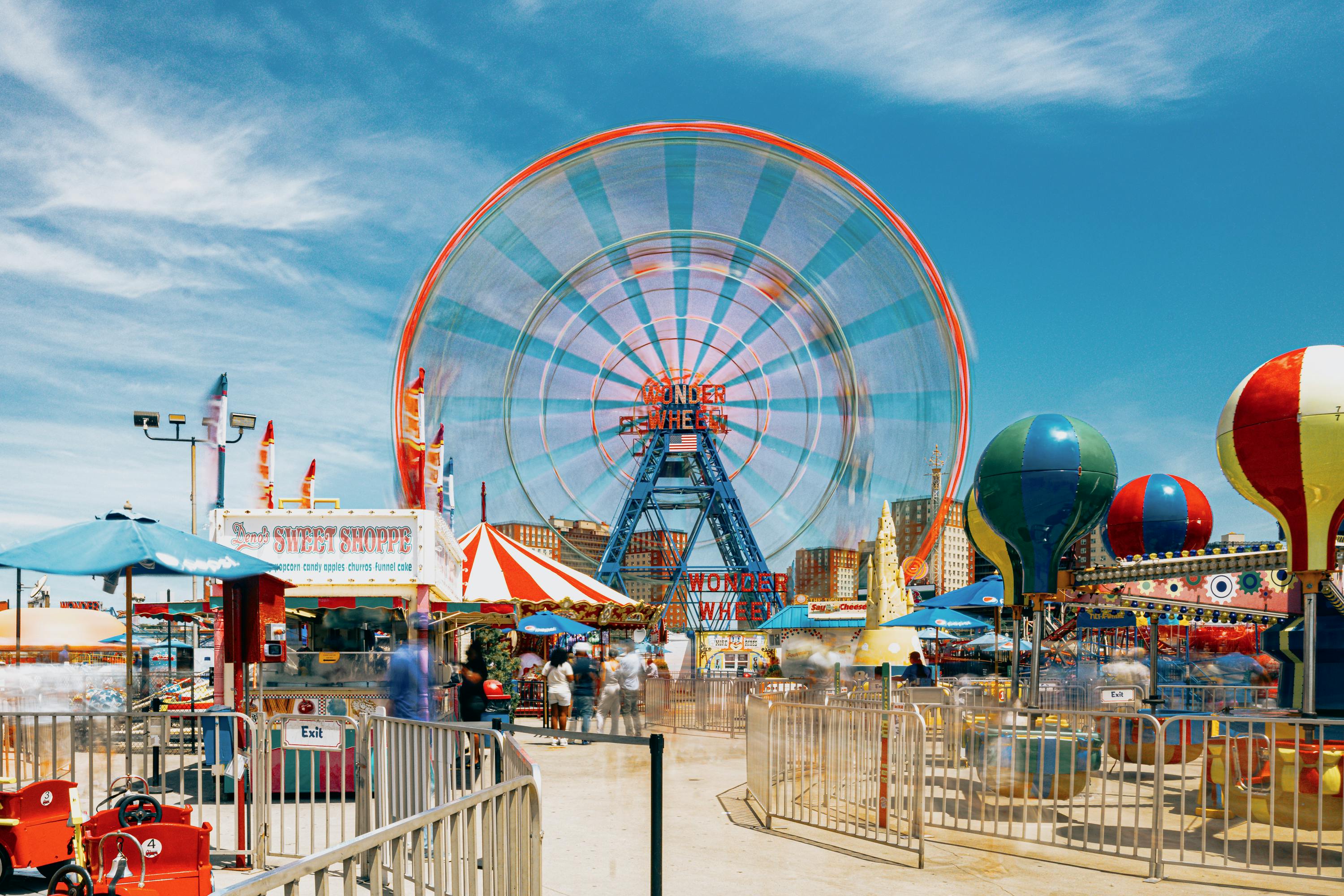
Entering the world of modeling can be both exciting and overwhelming. Preparing yourself for the unique challenges and opportunities ahead is crucial, especially with the evolution of **modeling techniques** in recent years. This article will guide you through essential steps and tips that will not only simplify the process of starting your modeling career but will also set you up for success in 2025 and beyond.
Understanding Different Modeling Techniques
It’s essential to familiarize yourself with a variety of **modeling techniques** available today. Knowing the advantages and best practices of different methods will empower you for practical applications in various fields. For example, insights from **statistical models** can enhance data analysis processes by providing interpretations from various datasets. Techniques like **linear regression** and **regression analysis** play critical roles in predictive analytics, allowing you to create models that forecast outcomes based on historical data.
Statistical Models and Their Importance
Statistical models, including **linear regression**, are fundamental tools in business and research for interpreting complex datasets. By utilizing these models, you can predict relationships between variables, which is indispensable across different industries such as finance and marketing. For example, a company can predict sales based on advertising expenditure using this technique. An understanding of **model evaluation** is equally necessary, as it measures the effectiveness of your predictions, reinforcing your model’s utility in real-world scenarios.
Embracing Predictive Analytics
Predictive analytics spurs remarkable enhancements in decision-making. Leveraging **machine learning models** allows users to tap into vast amounts of data, providing informed insights that drive strategies. A robust framework for **model selection** ensures that the appropriate model is utilized, thereby increasing the likelihood of accurate predictions. Consider developing a structured approach to testing various models to achieve the best performance metrics for your specific datasets.
Model Deployment and Real-Time Applications
Once you've created a model, understanding how to effectively deploy it in real-time scenarios is vital. The transition from theory to practical application involves the use of **data pipelines** for smooth processing. Integrating modeling software that aligns with your unique needs ensures streamlined operations, paving the way for insightful **data visualization** that communicates findings clearly to stakeholders.
Practical Steps for Model Deployment
Deploying a model typically encompasses multiple steps: selecting an appropriate deployment platform, integrating it with existing workflows, and conducting thorough **model testing**. Using **cloud modeling** solutions can essentially boost collaboration, enabling stakeholders to access models remotely. Additionally, employing **real-time modeling** techniques helps in dynamically responding to changing data and preserving relevance in your analyses.
Ensuring Model Scalability
As your modeling efforts grow, ensuring **model scalability** becomes critical for handling increased data loads without compromising performance. Through effective **model optimization**, you can enhance both efficiency and accuracy, particularly in **risk modeling** scenarios where decisions may have far-reaching consequences. Monitoring characteristics such as resource consumption and response time better prepares you for future demands and challenges, emphasizing the importance of continuous improvement and adaptation.
Advanced Techniques in Modeling
Diving into advanced modeling encompasses understanding vibrant techniques like **neural networks** and **decision trees**. For practitioners looking to refine their skills towards emerging methods, familiarizing yourself with **ensemble methods** can yield powerful predictive capabilities by leveraging the outcomes of multiple models for enhanced accuracy.
Exploring Machine Learning Models
Machine learning presents a myriad of opportunities for adept problem-solving. By incorporating algorithms such as **deep learning methods**, you can analyze and interpret vast datasets far beyond the realm of traditional software. Staying updated with how to implement cross-validation techniques ensures your models generalize well in various scenarios, preventing issues related to overfitting or underfitting.
Optimization and Model Diagnostics
Optimizing your model is a continuous task that assures accuracy and reliability. Focusing on parameters such as model performance metrics and diagnostics provides critical insights into how models interpret and respond to data inputs. Engaging in practices like sensitivity analysis furthers understanding of what influences results, confirming robustness against uncertainties in input data.
Key Takeaways
- Familiarize yourself with various modeling techniques such as statistical models and predictive analytics.
- Ensure effective model deployment and integration while paying attention to scalability.
- Be open to learning advanced modeling techniques like machine learning and deep learning methods.
- Prioritize **model diagnostics** and ongoing optimization to maintain model effectiveness.
- Consider ethical modeling practices that respect data privacy and integrity.
FAQ
1. What are some common modeling techniques beginners should learn?
For those venturing into the world of modeling, common techniques to understand include **regression analysis**, **linear regression**, and basic machine learning models. Familiarizing yourself with **data visualization** techniques will also enhance your ability to communicate models effectively to various stakeholders.
2. How important is model validation in predictive analytics?
Model validation is crucial in predictive analytics as it confirms that the model adequately represents real-world data and can reliably predict future outcomes. Techniques like cross-validation ensure that your model does not overfit the training data and maintains high correctness in future predictions.
3. What roles do modeling software play in the modeling process?
Modeling software facilitates efficient data analysis and modeling by providing tools for data preprocessing, model testing, and comparison of results. Such software streamlines the entire process, making it more accessible, especially to beginners, while allowing established professionals to optimize their workflows.
4. How do I ensure my models are scalable for future needs?
To ensure scalability, consider using cloud computing resources, designing adaptable data pipelines, and ongoing assessments of resource consumption. Maintaining flexible modeling approaches will aid in incorporating modifications smoothly as data demands increase.
5. What ethical considerations should I keep in mind while modeling?
Ethical considerations include respecting data privacy, utilizing transparent algorithms, and ensuring fail-safes to avoid biases. Prioritizing **ethical modeling** practices is essential for maintaining trust and integrity in decision-making processes influenced by your models.
6. Can you explain the significance of feature selection in models?
Feature selection is essential as it helps to improve model performance by reducing complexity, enhancing interpretability, and decreasing overfitting risks. Choosing relevant features ensures that the modeling process remains focused on the most impactful data, fostering clearer insights and actionable outcomes.
7. What is the difference between supervised and unsupervised modeling?
Supervised modeling involves training a model on labeled data, where the outcome is known, effectively allowing predictions. On the other hand, unsupervised modeling trains on unlabeled data, identifying patterns and clusters without prior knowledge of outcomes. Understanding both approaches enriches your capability in data analysis.